CBPF AI Group Meeting with Petrobras: Artificial Intelligence in Oil Exploration
The Laboratory of Computing and Artificial Intelligence (LITCOMP-AI) held the "CBPF AI Group Meeting with Petrobras: Artificial Intelligence in Oil Exploration - From Petrography to Seismics" on April 29, 2024. The event covered five main topics: AI in Nuclear Magnetic Resonance, AI in Well Log Analysis, AI in Seismic Data Exploration, AI in Petrographic Thin Section Characterization, and Sustainability in Computational Systems for AI.
AI in Nuclear Magnetic Resonance
Dr. Jorge Luis Gonzalez Alfonso from the Institute of Physics at UFES presented applications of AI in Nuclear Magnetic Resonance (NMR). He discussed using machine learning algorithms, such as convolutional neural networks and supervised learning methods, to identify complex patterns in NMR data. These algorithms can correlate visual characteristics of rocks with known magnetic properties, enabling the prediction of NMR spectra from rock images. This approach aims to reduce the need for direct experimental measurements, saving time and resources. Additionally, the accuracy of the models depends on the quality and quantity of training data, highlighting the importance of a robust and diverse dataset.
AI in Well Log Analysis
Dr. Clécio Roque De Bom from CBPF discussed using machine learning techniques in well log analysis. He explained how algorithms, including decision trees and neural networks, can interpret large volumes of geological and geophysical data. These techniques enable the identification of patterns and trends that are difficult to detect manually. Automated analysis can provide detailed information about the composition and structure of subsurface formations, aiding decision-making during oil exploration and production. Integrating these technologies promises to improve the efficiency and accuracy of well log analysis.
AI in Seismic Data Exploration
In another presentation, Dr. Clécio Roque De Bom addressed the application of machine learning in seismic data analysis. He detailed how machine learning models, such as deep neural networks and unsupervised learning methods, can be trained to detect patterns and anomalies in large seismic datasets. Seismic data analysis involves interpreting reflected seismic waves to map subsurface structures. AI models can improve the resolution and accuracy of these interpretations, facilitating the identification of oil and gas reserves. Moreover, automated seismic data analysis can speed up the exploration process, making it more efficient and less dependent on human intervention.
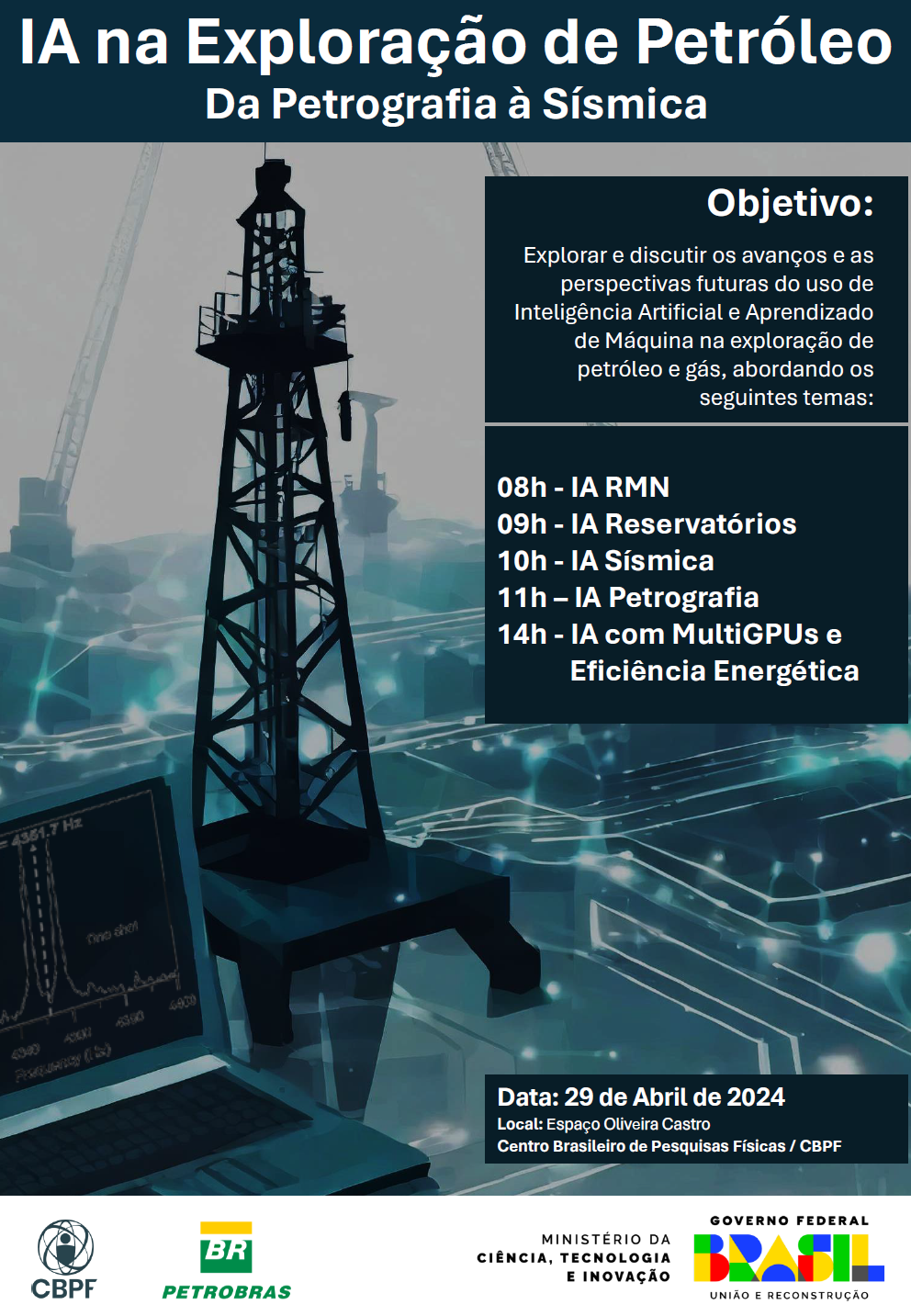
AI in Petrographic Thin Section Characterization
Dr. Elisangela Lopes de Faria from FACC/CBPF presented advances in petrographic thin section characterization using deep learning. She explained how convolutional neural networks could be trained to recognize and classify different types of rocks from microscopic images. This process involves image segmentation to identify specific geological features and classification algorithms to categorize rock types. Automating this method can increase the accuracy and consistency of petrographic analyses, reducing the time required for manual characterization. Accurate rock characterization is essential for reservoir evaluation and informed decision-making in oil exploration.
Sustainability in Computational Systems for AI
Dr. Marcelo Portes de Albuquerque from CBPF discussed implementing sustainable computational systems at the CBPF Artificial Intelligence Laboratory. He emphasized the importance of using AI technologies efficiently in terms of energy and environmental responsibility. The presentation covered using specialized hardware, such as high-performance GPUs and efficient cooling systems, to reduce energy consumption. Additionally, he discussed adopting green computing practices, including using renewable energy and optimizing algorithms to minimize the carbon footprint. Implementing these systems aims to improve the sustainability of AI operations, aligning with energy efficiency and emission reduction goals.
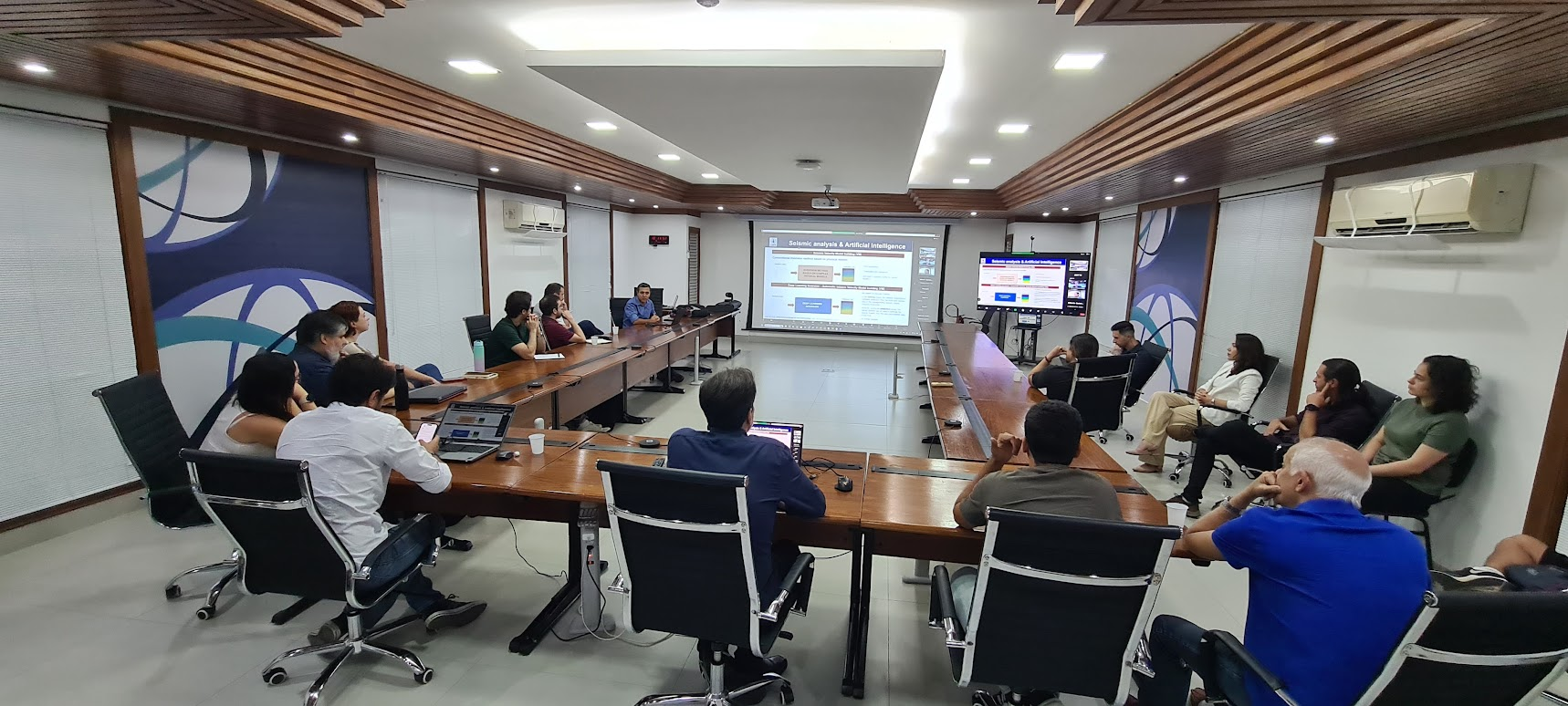
Roundtable Discussion - Innovative R&D Conversations Between Academia and Industry
In the event's final part, a roundtable discussion on collaboration between academia and industry was moderated by Dr. Marcelo Portes de Albuquerque and Dr. Bernardo Coutinho from Petrobras. They encouraged all attendees to participate in the conversation.
The discussion highlighted the importance of R&D partnerships in promoting technological innovation in oil exploration and production, focusing on AI applications. The need for an integrated and multidisciplinary approach to address industry challenges was emphasized, underscoring continuous collaboration as essential for progress.
One central topic was defining and aligning deliverables in research projects, which face challenges due to constant changes during development. The partnership with Petrobras has been crucial in this aspect, with ongoing discussions to align expectations and objectives between teams. The acceptance of delivering results in library format was seen as a significant advancement, demonstrating the feasibility of this approach in ongoing R&D projects.
Another significant theme was the computational infrastructure required for AI, including HPC systems with multi-GPUs. The proposal for visits to computers and associated training highlighted the importance of this infrastructure for the projects. The construction of this infrastructure by the Brazilian group was positively received, showcasing the local potential to develop high-performance AI solutions.
This debate reaffirmed the importance of continuous collaboration between academia and industry to address the technological challenges of the oil sector, promoting significant advances through AI integration and other innovations.