CBPF Team Wins International Strong Gravitational Lensing Challenge
The LITCOMP-IA team from CBPF has won the second Strong Gravitational Lensing Challenge with machine learning, showcasing Brazil's potential in astrophysics and paving the way for new scientific applications.
The Brazilian Center for Research in Physics (CBPF), through the LITCOMP-IA team, has emerged victorious in the second Strong Gravitational Lensing Challenge, an international competition that brings together experts in astrophysics and data science. The challenge involved identifying strong gravitational lensing systems in a set of simulated astronomical images using advanced machine learning and artificial intelligence techniques.
The Strong Gravitational Lensing Challenge was organized by the Bologna Lens Factory team, a research group focused on astrophysics at the University of Bologna, Italy. This group is renowned for its significant contributions to the study of gravitational lenses and cosmology and organized the challenge as a way to stimulate the development and application of advanced data analysis techniques, including machine learning and artificial intelligence, to identify gravitational lensing systems in large astronomical datasets.
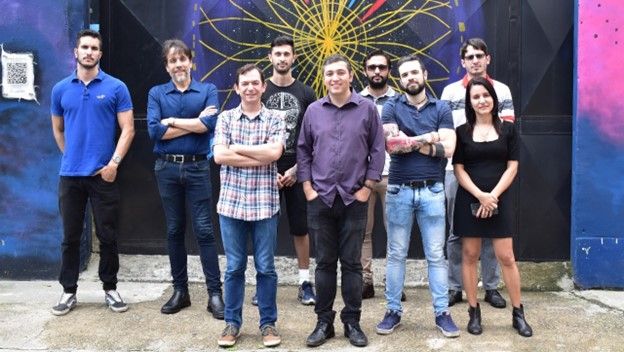
From left to right: Patrick, Marcelo, Martín, Paulo, Clécio, Pedro, Manuel, Marcos e Luciana
The Winning Team
The winning team of the challenge, self-named CAST (CBPF Arc Search Team), consists of
Team Leader
- Clécio De Bom - CBPF e CEFET-RJ
Technological Development
- Patrick Schubert - CBPF
- Manuel Blanco Valentín - CBPF
- Luciana Oliva Dias - CBPF
- Marcelo Portes de Albuquerque - CBPF
- Paulo José Russano - CBPF
- Marcos Vinicius Silva - CBPF
- Pedro Russano - CBPF
Scientific Research
- Cristina Furlanetto - UFRGS
- Martín Makler - CBPF
All team members work in the fields of Artificial Intelligence, Computer Vision, and/or Cosmology. In addition to winning 1st place, CAST also secured the 3rd, 4th, and 6th places.
The Phenomenon of Strong Gravitational Lensing: Strong gravitational lenses occur when the light from distant astronomical objects, such as galaxies or quasars, is bent and amplified by a large concentration of mass (such as another galaxy or galaxy cluster) located between the observed object and the observer. This phenomenon not only enables the observation of distant objects and the investigation of the universe's structure but is also essential for the study of dark matter, which does not emit light and therefore cannot be observed directly.
Strategies and Methodologies of the Winning Team: The CBPF team developed a winning strategy involving the use of deep neural networks to analyze and classify the images. One of the key innovations was the implementation of a network architecture with two branches, capable of processing images at different resolutions. This approach allowed for a more detailed and accurate analysis of the images, significantly improving the ability to identify gravitational lensing systems.
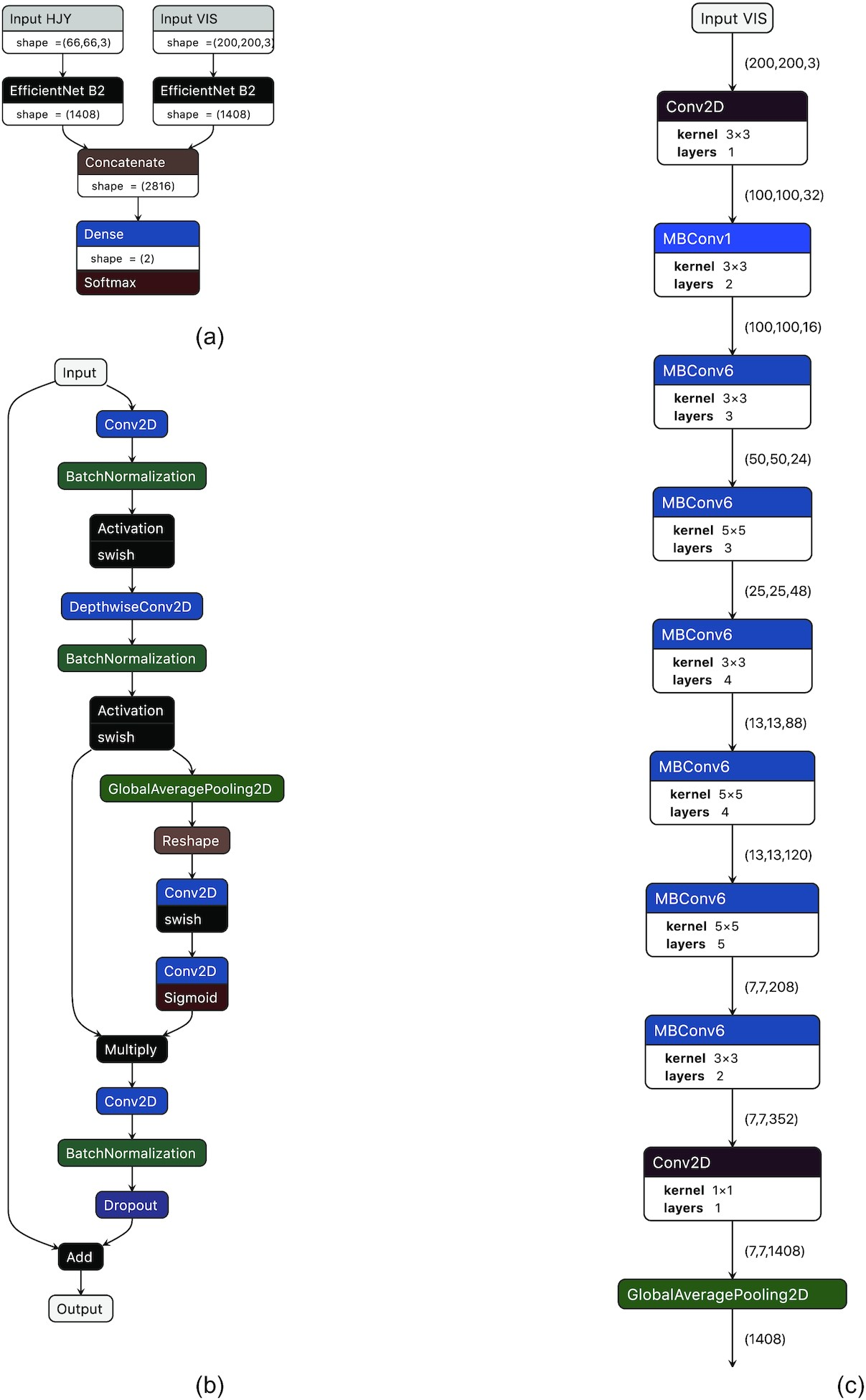
The deep learning architecture used in this work was based on the EfficientNet-B2 model. Panel (a): our two-branch model, used to simultaneously train images in the H, J, Y (Near Infrared) and VIS (Visible) bands. Panel (b): Mobile Inverted Bottleneck Convolution block, with compression and excitation phases. Panel (c): complete architecture of the EfficientNet-B2 model. The MBConv blocks are essential for the model's structure, acting as an efficient mechanism to capture image features through a combination of compression and excitation within the learning process. This configuration allows the model to dynamically adjust the importance of different learned features during training, optimizing the accuracy in identifying gravitational lenses.
MBConv, or Mobile Inverted Bottleneck Convolution, is a fundamental block used in deep neural network architectures, especially in the EfficientNet model family. This block is an enhanced variation of the traditional convolution module, designed to increase computational efficiency and the model's effectiveness in image processing tasks.
Results:
The victory of the LITCOMP-IA team from CBPF in this challenge highlights the capability and excellence of Brazilian scientific research in highly specialized fields such as astrophysics and data science. Furthermore, the models and methodologies developed as part of the challenge have the potential to be applied in other areas of astronomy and beyond, expanding the impact of this achievement.
Results:
The victory of the LITCOMP-IA team from CBPF in this challenge highlights the capability and excellence of Brazilian scientific research in highly specialized fields such as astrophysics and data science. Furthermore, the models and methodologies developed as part of the challenge have the potential to be applied in other areas of astronomy and beyond, expanding the impact of this achievement.
The achievement of the CBPF team in the second Strong Gravitational Lensing Challenge is a testament to the potential of interdisciplinary collaboration between astrophysics and data science. By applying advanced artificial intelligence techniques to astronomical research, the team not only secured a significant victory in a competitive international setting but also contributed to the advancement of scientific knowledge, opening up new possibilities for exploring the universe.
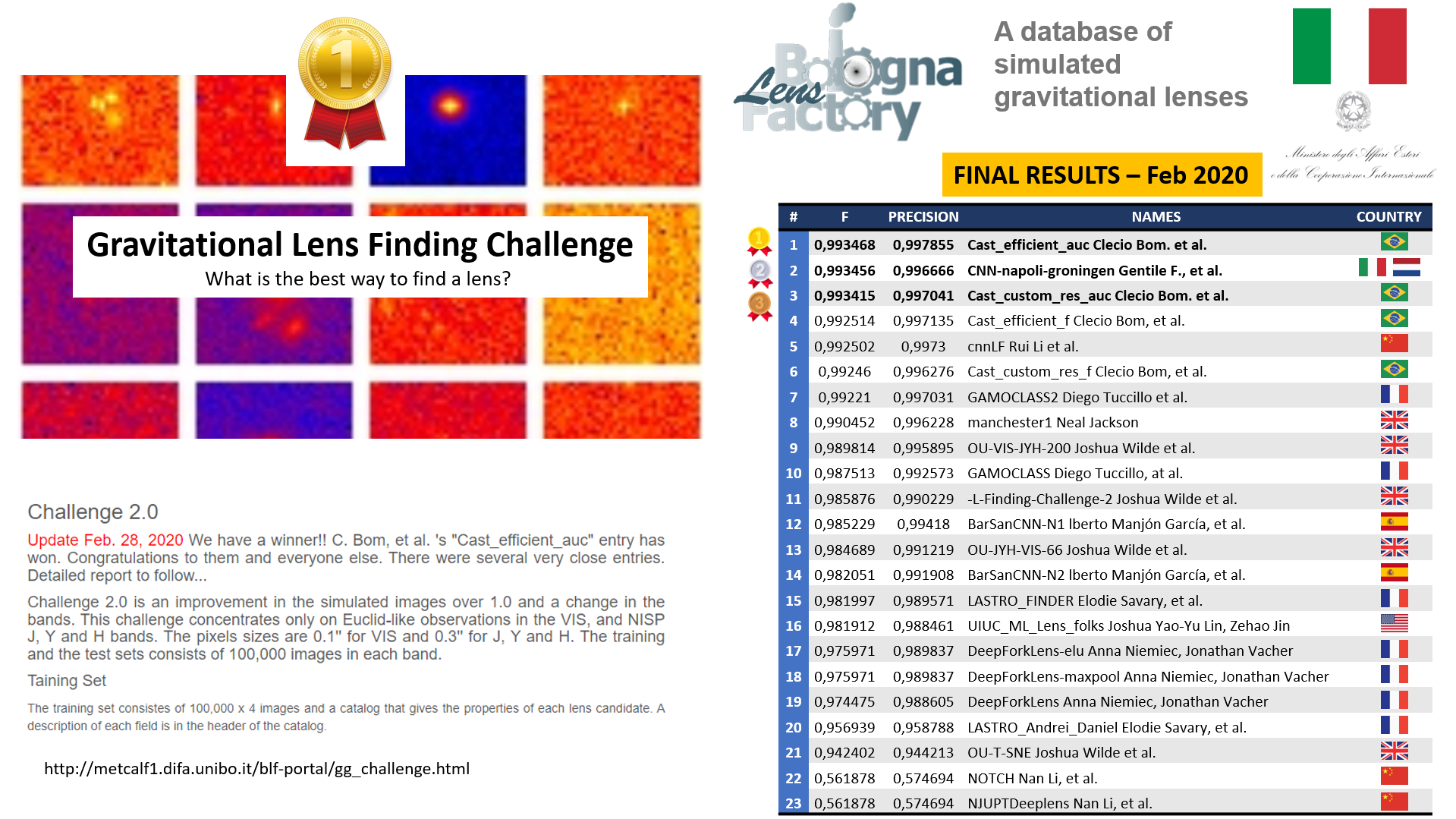
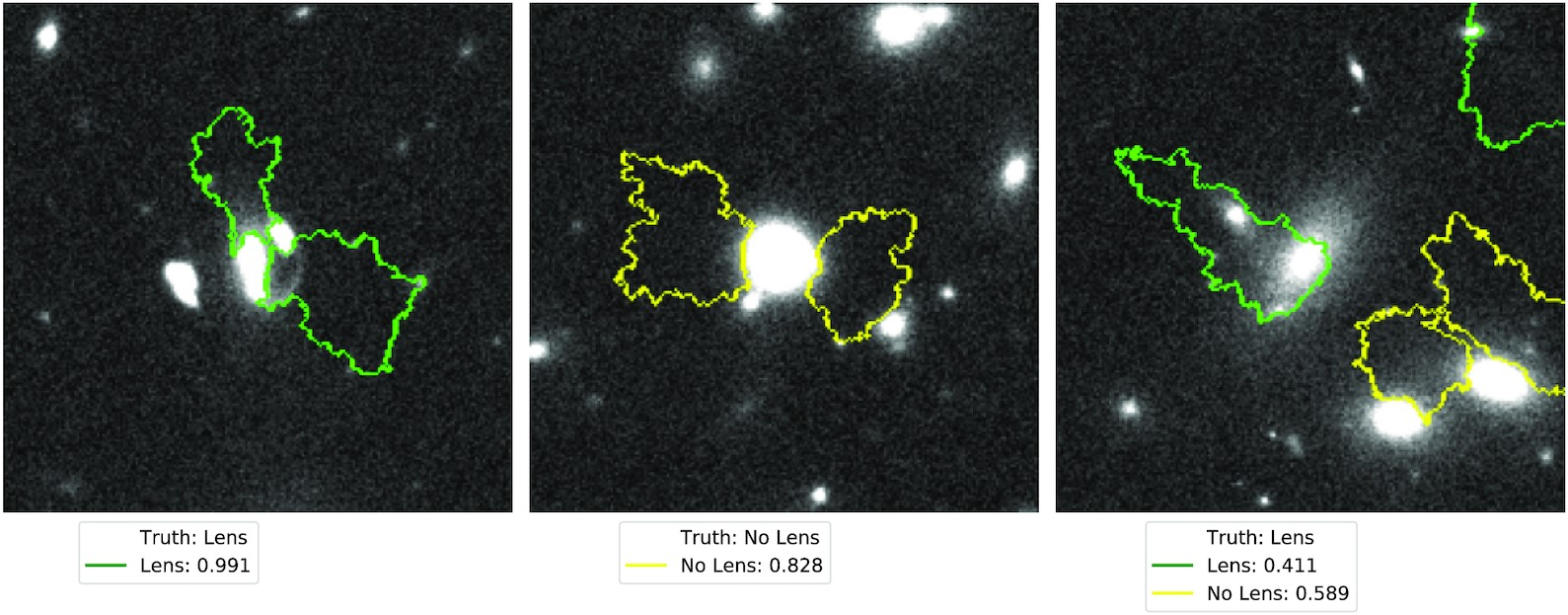
For more details:
Developing a victorious strategy to the second strong gravitational lensing data challenge
C R Bom, B M O Fraga, L O Dias, P Schubert, M Blanco Valentin, C Furlanetto, M Makler, K Teles, M Portes de Albuquerque, R Benton Metcalf Monthly Notices of the Royal Astronomical Society, Volume 515, Issue 4, October 2022, Pages 5121–5134,
https://doi.org/10.1093/mnras/stac2047Published: 22 July 2022 Article history
ABSTRACT
Strong lensing is a powerful probe of the matter distribution in galaxies and clusters and a relevant tool for cosmography. Analyses of strong gravitational lenses with deep learning have become a popular approach due to these astronomical objects’ rarity and image complexity. Next-generation surveys will provide more opportunities to derive science from these objects and an increasing data volume to be analysed. However, finding strong lenses is challenging, as their number densities are orders of magnitude below those of galaxies. Therefore, specific strong lensing search algorithms are required to discover the highest number of systems possible with high purity and low false alarm rate. The need for better algorithms has prompted the development of an open community data science competition named strong gravitational lensing challenge (SGLC). This work presents the deep learning strategies and methodology used to design the highest scoring algorithm in the second SGLC (II SGLC). We discuss the approach used for this data set, the choice of a suitable architecture, particularly the use of a network with two branches to work with images in different resolutions, and its optimization. We also discuss the detectability limit, the lessons learned, and prospects for defining a tailor-made architecture in a survey in contrast to a general one. Finally, we release the models and discuss the best choice to easily adapt the model to a data set representing a survey with a different instrument. This work helps to take a step towards efficient, adaptable, and accurate analyses of strong lenses with deep learning frameworks.